Machines + Society #13: Stagnant questions; Inflamed Ladies; Reverse microscopy
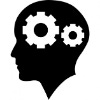
machines + society
Mako Shen | Aug 31, 2020
Before the essay, here are the recall questions from last month:
What, specifically, is the problem with how we consume media now if we want to learn?
What are some dimensions to consider if we want machines to augment our ability to remember?
Questioning Our Way to Progress
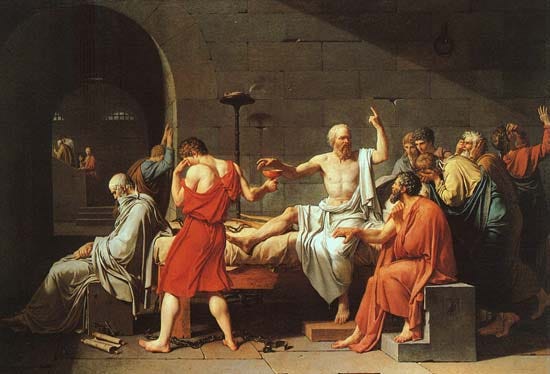
[Louis-David’s The Death of Socrates, via Wikipedia]
There is growing evidence for scientific stagnation. In this essay, I explore the link between scientific incrementalism and stagnation. I then describe a problem-formulation methodology that I believe encourages more extraordinary (in the Kuhnian sense) research, and make some practical suggestions about using questions.
I. Stagnation
Are ideas getting harder to find? Maybe [1]. A recent paper by the Economist Nicholas Bloom and colleagues argues persuasively that in domains ranging from energy to medicine, we are getting far less use out of each additional resource (lab equipment, researchers, year of education) that we put in. The most vivid example they give is the case of Moore’s law: it now takes 18 times more researchers to double chip density compared to the 1970s. This trend is consistent across the natural sciences, agricultural and medical technology, and at an aggregate level across the entire U.S. economy.
This essay isn't an investigation into scientific stagnation. Instead, I'm going to focus on a particular factor in the decline of scientific progress: scientific incrementalism. It's also referred to as 'gap spotting'. By scientific incrementalism, I mean the emphasis on making small contributions to existing modes of thought. You see this in papers that apply the most recent computer vision algorithm to the task of text recognition/landslide detection/crowd counting. Contrast this with research that significantly departs from previous frameworks of thought. One classic example is the departure of the theory of general relativity from Newtonian mechanics. Thomas Kuhn talked about normal science versus extraordinary science [2]; scientific incrementalism is the belief in the dominance of normal science.
II. Scientific Incrementalism
Like others, Ed Boyden, the famous neuroscientist, has several crazy ideas. Unlike others, his ideas have radically changed how people research neuroscience. He is known best for his work in optogenetics, which lets you essentially add an on-switch to individual neurons (this led to his award of the Breakthrough Prize for "transformative advances toward understanding living systems").
One of the other crazy ideas he's had is expansion microscopy. In neuroscience it is really hard to examine individual neurons; the diffraction limit means that microscopes can only view cells as small as a few hundred nanometers, far larger than the size of most biological molecules. Ed Boyden notices that, rather than trying to increase the resolution of the microscope, it's possible to expand the neurons themselves. He had the wicked idea of using the absorptive polymer from baby diapers to increase the volume of the cells by over 100 times.
But he couldn't get funding. In his words, "people thought it was nonsense. People were skeptical. People hated it. Nine out of my first ten grants that I wrote on it were rejected. If it weren’t for the Open Philanthropy Project that heard about our struggles to get this project funded — through, again, a set of links that were, as far as I can tell, largely luck driven — maybe our group would have been out of business."
The crazy thing? This was four years ago; after he had won widespread recognition and admiration for revolutionizing neuroscience.
Meanwhile, journals and grants are by and large filled with and awarded to incremental research. Several scholars, most notably Alvesson and Sandberg [3], have examined the diversity of research in a variety of fields ranging from genetics [4] to information systems [5]. They find that the predominant mode of research is gap-spotting. Our institutions are largely driven by scientific incrementalism.
Incrementalism as the default mode of research is problematic for two big reasons (among others).
First, scientific incrementalism as the standard of research has a chilling effect on unusual ideas. To understand this, we have to understand the academic ladder and the incentives that drive it.
The goal for most anyone who wants to remain in academia is tenured professorship. This means that your pay is guaranteed and you have the freedom to publish whatever you would like.
To get to this highly prized position there are several hoops you have to jump through. First, you need to get a PhD. Where I studied computer science, most of my classmates who ended up in the best graduate programs had publications in top conferences (NeurIPS/ICML) or journals (PNAS, Nature). If you are accepted into a PhD program, you are expected from the second year onward to publish frequently. This intense 'publish or perish' mindset continues through your postdoctoral research position, to the assistant professorship, and only lightens up when you become a tenured professor. Then, as a tenured professor with many many publications in journals, you can then become the gatekeeper of such journals, and decide which papers are submitted.
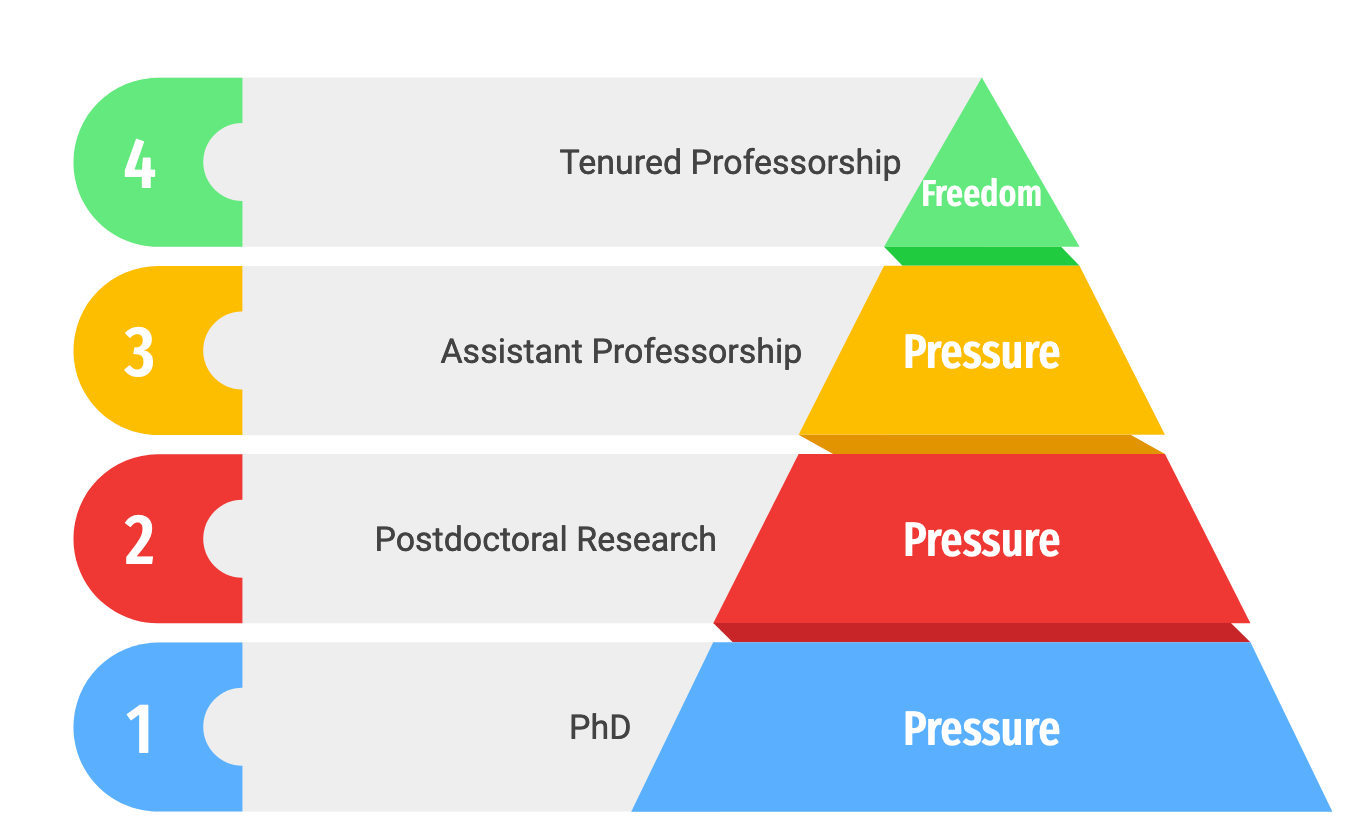
A pyramid diagram I made illustrating the progress of research scholars within academia.
The chilling effect on unusual research comes in two forms: top-down and bottom-up.
The top-down perspective is that of journal editors. They are often tenured professors who have spent most of their careers pumping out incremental research. Then when an editor receives a paper that challenges prior assumptions, there is a good chance that the editor's own research is called into question. People tend to reject information that questions their existing beliefs. Scholars are no different; they will reject the radical paper. Boyden also talks about another symptom: the recent extent of specialization has rendered most researchers unable to evaluate proposals that draw from a range of different fields.
The bottom-up perspective comes from the aspiring professors. As a non-tenured academic, you will want to publish as many papers in quality journals as you can to increase the chances of tenure. This means conforming to the expectations of journal-editors. So forget about your crazy idea of using diaper polymers to create giant bloated neurons. Instead, just publish something about using CRISPR on a new type of cell.
These two pressures reinforce each other and make truly groundbreaking forms of research increasingly less likely.
Second, incremental research systematically under-problematizes a field. For instance, imagine you're researching how social priming (e.g. unscrambling adjectives that have to do with old-age) influences your shopping habits. You can do absolutely everything correctly— rigorous statistical analysis, sample size large enough, representative samples, et cetera— and still be wrong because social priming is false [6]. Yet by conducting your research and reporting your p-value of <0.001, you are implicitly assuming that the methodology informed by prior research is completely solid.
Your experiment means nothing if it is based on incorrect work. And because incremental research assumes much more than extraordinary research, it is, from an outside view, more likely to be false.

(This stylized pair of equations leave out many important details but capture the intuition for the second point.)
Of course, incremental research has an important its place in providing more solid grounding to existing theories. A world where all research attempted to be extraordinary would be deeply problematic in other ways. Right now, however, if extraordinary people like Ed Boyden (and there are a number of similar accounts to his) struggle this much to get funding, we have a problem. The balance needs to shift towards more inventive research.
III. Uncovering Assumptions
Suppose you are a researcher that is convinced that scientific incrementalism is a problem, and you want to do some extraordinary research. What do you do?
Luckily, Alvesson and Sandburg (2011) have you covered. They have a metaprocess that looks something like this:
1) Identify a domain of literature for close investigation
2) Identify, articulate, then evaluate the set of assumptions within that domain.
3) Generate then evaluate alternative explanations for the assumptions.
While this process seems obvious, to me the key insight was the importance of articulating assumptions.
Assumptions are submodules of thought within existing research. By nature, they are seldom explicit. Uncovering the assumptions behind a statement is hard. Perhaps much harder than one would think.
Take for instance, the equation 1+3 = 4. We all consider this valid because we were taught that 1+1 = 2 and we know that the 4 comes after 3, which comes directly after 2. But what about the assumptions behind why 1 + 1 = 2? To validate these assumptions Then we need to understand the Paeno axioms of reflexivity, transitivity, induction, etc. These are deeper concepts which then open up a new avenue of inquiry.
It's difficult to actually state the implicit assumptions behind a piece of research because that requires a detailed model of the reasoning machinery of a field. I would venture to say a good litmus test for deep understanding of a field is an ability to articulate its assumptions.
But how do we start thinking about assumptions? Alvesson and Sandburg have some pretty good ideas. They present five major types of assumptions:
In-house assumptions: typically accepted within a particular school of thought (but potentially questioned outside of it). For instance, the great man theory of history holds that history can be largely explained by particular great men (think Hitler, Bonaparte). If you were to claim that great men are really just a product of their social environment, you would be challenging an in-house assumption. These are typically the easiest assumptions to identify.
Paradigmatic assumptions: slightly broader than in-field assumptions and are core to the reasoning of a particular field. For example, revealed preference theory, which assumes that the preferences of consumers can be accurately inferred from their purchasing habits, is a paradigmatic assumption within economics.
Field assumptions: assumptions that are shared across fields and are even t broader than paradigmatic assumptions. A notable example of a successful challenge of a field assumption came with Herbert Simon's notion of 'bounded rationality'. Neoclassical economics initially assumed people could be faithfully modeled as infinitely rational agents with unlimited cognitive resources; Simon called this assumption into question and proposed the inclusion of cognitively limited agents.
Root metaphors: the visual imagery and metaphors shared within a particular field. For instance, macroeconomists and their disciples often talk about the economy using the metaphor of a machine (which many have condemned). Paul Krugman, for instance, has said that what drew him to economics was "the beauty of pushing a button to solve problems."
Ideological assumptions: these are the political, societal, and moral assumptions. For instance, the great man theory of history also contains the implicit assumption that history is largely influenced by men. Subsequent accounts of history have emphasized the role of women, challenging this male-centric ideological assumption.
In-house, field, and paradigmatic assumptions can be grouped as different depths of implicit knowledge. Root metaphors are about images and analogy, and ideology is a more direct reflection of the private worldview of the scientists.
Here is one instance where I've identified an assumption and am considering an alternative:
The 'agent' assumption is a field assumption shared across economics, cognitive science, computer science, and sociology.
Roughly, it states that people can be well modeled as having fixed objectives.
How could we move beyond this? Can we start to think of people as ecosystems, akin to the Buddhist perspective on selfhood?
This line of questioning is also part of the Center for Human-Compatible AI’s latest research agenda.
Of course, better research questions alone will not fix the scientific ecosystem. Yet I’m convinced this type of thinking could come in useful by making extraordinary research slightly more probable.
IV. Questioning Further
I said before that stating assumptions is a good litmus test for deep understanding of a field. I think the ability to articulate the good questions within your field is equally important.
Richard Hamming in a famous speech recounts his experience at Bell Labs. He makes a point of going to the various tables of mathematicians, chemists, physicists, and asks them the same question: "what are the most important problems in your field?" Hamming’s conclusion was that the scientists who succeeded were the ones who were most willing to spend time considering difficult questions like these.
Yet I haven't found much in the way of thinking about how questions can be used to better foster research. Outside of the Alvesson and Sandburg paper [3], I see very few attempts at systematizing the use of questions.
After recently surveying literature in different fields, I've concluded that it would be really helpful to have better norms around using questions to drive inquiry.
First, I think survey papers should include the central questions that their particular subfield is trying to answer. One great recent example is The Malicious Use of Artificial Intelligence: Forecasting, Prevention, and Mitigation [7]. The authors clearly lay out the motivating question for the paper, and enumerate a large number of questions to guide further research. I have a clear sense of the landscape of research and the type of thinking required to tackle interesting problems.
Second, more researchers should have explicit questions of interest listed on their webpage. Fiery Cushman of the Harvard Moral Psychology Lab has a great example of this on his lab website. For instance, Cushman’s 2015 paper ‘Deconstructing intent to reconstruct morality’ [8] makes a lot more sense when understood as a specific angle on the question, ‘How do we judge people who cause harm?’. For labs, these guiding questions provide a scaffold that is really useful to contextualize specific pieces of research.
All this questioning is certainly not limited to academic research. Why not try this approach to orient your personal syllabus? For a challenging but rewarding task, try to articulate your guiding questions within a specific field. For example, if you are interested in cognitive science, you might ask “what is most convincing about the dual process theory of cognition?”, “what type of behaviors is the dual process model best at explaining?”, “how could we apply the dual process model within organizational psychology?”
Recall questions:
What's wrong with the current trend of incremental research? Give three reasons.
How might you break down the three useful distinctions in types of assumptions? Can you give examples?
EDIT (9/1/20):
80k hours just released an excellent list of research questions in several different fields.
References:
[1] Bloom, Nicholas. “Are Ideas Getting Harder to Find?” THE AMERICAN ECONOMIC REVIEW 110, no. 4 (2020): 41.
[2] Bird, Alexander, "Thomas Kuhn", The Stanford Encyclopedia of Philosophy (Winter 2018 Edition), Edward N. Zalta (ed.), URL=<https://plato.stanford.edu/archives/win2018/entries/thomas-kuhn/>.
[3] Sandberg, J., & Alvesson, M. (2011). Ways of constructing research questions: gap-spotting or problematization? Organization, 18(1), 23–44. https://doi.org/10.1177/1350508410372151
[4] Sverdlov, E.D. Incremental Science: Papers and Grants, Yes; Discoveries, No. Mol. Genet. Microbiol. Virol.33, 207–216 (2018). https://doi.org/10.3103/S0891416818040079
[5] Barrett, Michael, and Geoff Walsham. “Making Contributions From Interpretive Case Studies: Examining Processes of Construction and Use.” In Information Systems Research: Relevant Theory and Informed Practice, 293–312. IFIP International Federation for Information Processing. Boston, MA: Springer US, 2004. https://doi.org/10.1007/1-4020-8095-6_17.
[6] R, Dr. “Reconstruction of a Train Wreck: How Priming Research Went off the Rails.” Replicability-Index (blog), February 2, 2017. https://replicationindex.com/2017/02/02/reconstruction-of-a-train-wreck-how-priming-research-went-of-the-rails/.
[7] Brundage, Miles, Shahar Avin, Jack Clark, Helen Toner, Peter Eckersley, Ben Garfinkel, Allan Dafoe et al. "The malicious use of artificial intelligence: Forecasting, prevention, and mitigation." arXiv preprint arXiv:1802.07228 (2018).
[8] Cushman, Fiery. “Deconstructing Intent to Reconstruct Morality.” Current Opinion in Psychology 6 (December 2015): 97–103. https://doi.org/10.1016/j.copsyc.2015.06.003.
📰 Assorted Links 📰
The Strategic Consequences of Chinese Racism— "racism informs their view of the United States. From the Chinese perspective, the United States used to be a strong society that the Chinese respected when it was unicultural, defined by the centrality of Anglo- Protestant culture at the core of American national identity aligned with the political ideology of liberalism, the rule of law, and free market capitalism. The Chinese see multiculturalism as a sickness that has overtaken the United States, and a component of U.S. decline." From a 2013 Pentagon report. The whole thing is a pretty fascinating Chinese cultural overview. (h/t Gwern)
A million Chinese civilians were sent to live in Uyghur homes to surveil and ‘educate’. This is crazy.
Joke recommendation using dimensionality reduction and O(1) nearest neighbor clustering.
Apple, Epic, and the App Store. The best evaluation of App Store antitrust issues I have come across. Very readable.
Amazon removed a non-disparagement clause that was originally in its podcast software free-use agreement. While its somewhat reassuring to see the response to backlash, it’s frankly ridiculous to have had such a clause in the first place. +1 karma journalists.
GPT-3 Creative Writing Samples. “Even as I stare between wonder and fear,/The shapes thin to vapor; a hand grinds the sand,/And a cloud of dust spouts skyward point on point.”
Is experimental statistical evidence invalid because of idea correlation between researchers?
Miscellaneous
The syllabus for a graduate Behavioral Economics class.
The logistic map function models phenomena ranging from heartbeats during fibrillation to the irregular dripping of a tap. A simple video explanation.
*College as an incubator of Girardian terror. [H/t JL]
Meaningness appetizer. Explains why eternalism, materialism, mission, nihilism, and existentialism are five confused attitudes towards meaning.
Productivity update: for those that were intrigued by my Tools for Thinking article, I've updated my personal knowledge management system:
I have a large list of all the media that I want to consume (essays, books, films, lectures) in a page within Roam.
Every time I come across something that makes me think, I create a new note (tagged with metadata) summarizing the thoughts, with a few of my own questions sprinkled in. If I think that particular fact is worth dedicating 10 minutes to memorizing over my lifetime (from Michael Nielsen's Anki post), then I turn it into a flashcard within Remnote.
Every morning, I spend 10 minutes reviewing/adding flashcards within Remnote. I've found this to be the most difficult habit to start, but so far, I think it's really made a difference. I remember so much more of the material I read a month ago that I would consider me right now to have an unfair competitive advantage over myself 3 months ago in terms of knowledge acquisition.
Movie of the month
“Do not regret. Remember.” [H/t JZ]
🎧 Music 🎧
Foxwarren — Sunset Canyon
Yin Yin — Thom Kï Kï
Gnarls Barkley — Crazy
John Hartford — I Forgot to Forget